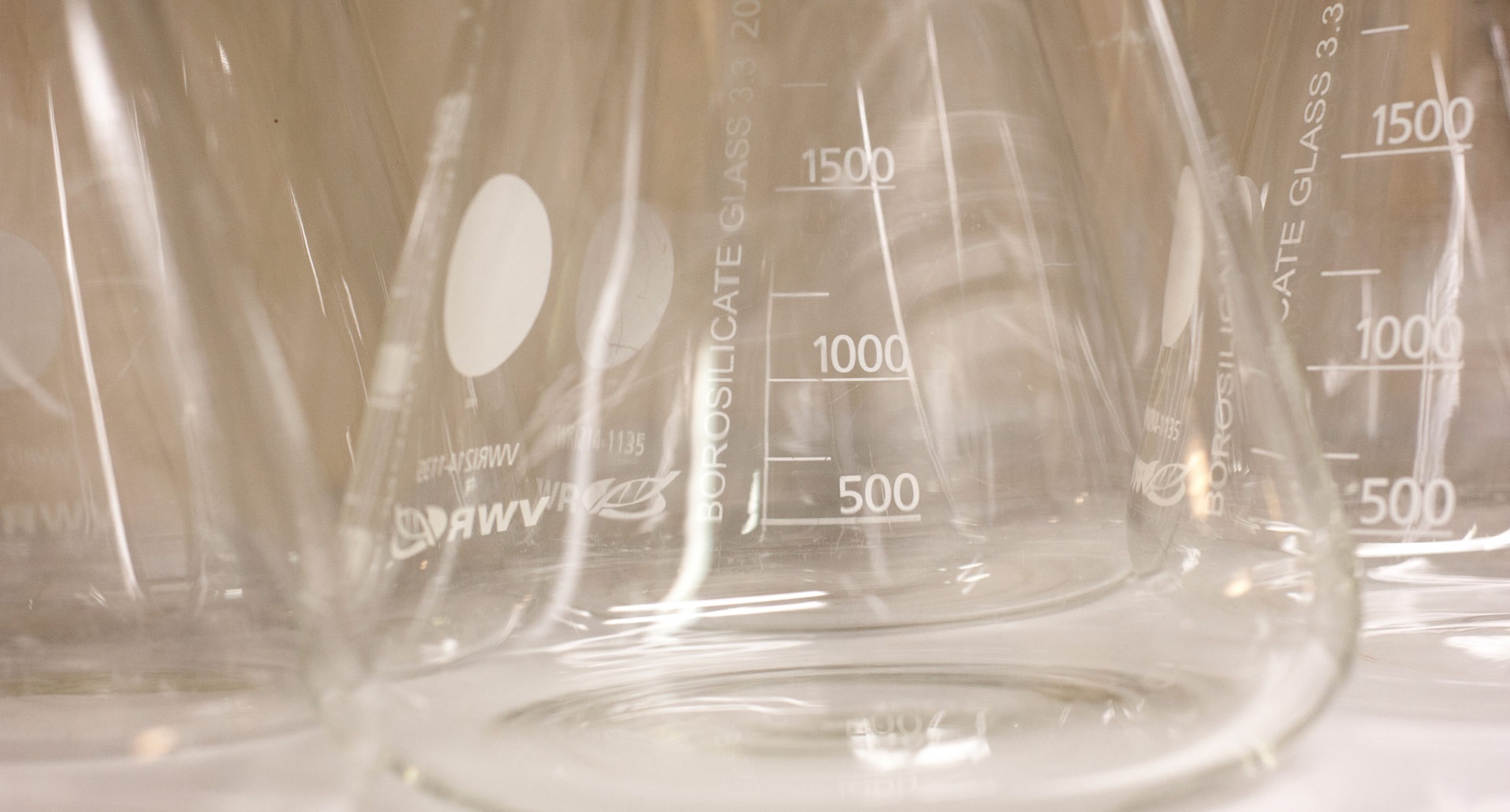
Closed: Determining the role of the adaptive immune system in controlling breast cancer metastasis and evolution
Project background
Immune dysregulation is a hallmark of cancer. We have shown that breast tumours genomically co -evolve with the immune response, resulting in immunoevasion (Sammut et al., Nature Immunology 2024; Arruda and Sammut et al., Cell Reports 2019). The interplay of tumour-immune coevolution and how this results in immunoevasion, particularly in the context of metastasis to immune-rich organs such as lymph nodes, remains a critical but poorly understood aspect of cancer biology.
This is particularly important in an aggressive subtype of breast cancer called triple-negative breast cancer (TNBC; negative for oestrogen, progesterone and HER2 receptors), which is frequently diagnosed in younger women and is characterised by high rates of lymph node metastasis, limited therapy options, and poor prognosis. TNBC is treated with chemo-immunotherapy, however around 40% of patients show a poor response to this (Schmid et al., NEJM, 2020) and have a poor prognosis. The mechanisms behind resistance and response to immunotherapy in TNBC are unknown, and therefore immunotherapies continue to be given in a one size fits all approach.
This proposal aims to unravel the biology underlying adverse immune evolution that results in failure of immunosurveillance and metastasis to immune-rich lymph nodes. In this project, you will leverage the laboratory’s expertise in translational cancer medicine as well as the generation and analysis of large-scale molecular profiling data from women with breast cancer, and its integration using machine learning (Sammut et al., Nature 2022), to generate a single-cell atlas of primary TNBCs and metastatic lymph nodes. By developing methods that combine this data, you will elucidate how the interaction between tumour cells, their microenvironment and the adaptive immune response enables immunoevasion during treatment with chemo-immunotherapy, resulting in therapy failure. You will determine biological mechanisms associated with response and resistance to immunotherapy and use this knowledge to identify new targets for which novel immunotherapies can be developed.
Project aims
- Identify the molecular alterations TNBC cells acquire to avoid immune recognition
- Characterise how the immunosuppressive tumour microenvironment facilitates immune escape and enables resistance to immunotherapy
- Using single cell B and T cell receptor sequencing, investigate how adaptive immune failure occurs and how immunotherapy restores immune function
Further details & requirements
This is a computational biology PhD project and you will be working in a multidisciplinary environment. You will be expected to have a background in computational biology or other quantitative subject. You will be working closely with experimental scientists, functional and basic immunologists (Melcher, Arwert, Centre for Translational Immunology), experts in tumour evolution (Graham, Centre for Evolution and Cancer) as well as members of the oncology multidisciplinary team at the Royal Marsden Hospital.
In this project we will analyse paired primary breast tumour and metastatic lymph node samples from patients newly diagnosed with TNBC recruited to the BELIEVE breast cancer study at the Royal Marsden Hospital.
We will generate bulk whole genome sequencing data and perform single cell RNA and B and T cell receptor sequencing (scRNA-seq; 10x Genomics) on all samples. WGS will establish the overall tumour genomic landscape, while scRNA-seq will identify and quantif y tumour, stroma, and immune cells in each sample. In the first part of the project, we will focus on identifying molecular alterations TNBC cells acquire to avoid immune recognition. We will use DNA sequencing to characterise the bulk mutational and copy number landscape in primary TNBCs and identify immuno-evasive genomic alterations associated with metastasis. We will refine this data further using scRNA-seq data and derive single cell signatures associated with immunoevasion. Hence, this approach will allow us to derive tumour-cell specific genomic and transcriptomic signatures associated with immune escape and failure of immunotherapy. We will then use ensemble machine learning methods to combine this data and pinpoint the exact combination of immunoevasive molecular alterations required to enable metastasis.
In the second part of the project, we will focus on characterising the role of the microenvironment in facilitating immune escape. Using the scRNA-seq data we will identify microenvironment cell types and states associated with immune evasion and reconstruct their differentiation trajectory to identify the key microenvironmental steps and molecular pathways associated with metastasis and determine whether tumour cells actively drive these changes. If so, this will provide evidence that tumour cells manipulate their microenvironment to enhance immunosuppression and facilitate metastasis and diversification. We will also infer cell-cell communication networks from scRNA-seq data to elucidate how microenvironmental cell types associated with immune evasion interact to establish an immunosuppressive microenvironment and validate these using spatial transcriptomic analyses.
Finally, we will use B and T cell receptor sequencing data to investigate the role of adaptive immune failure in enabling metastasis. We will develop novel B and T cell receptor biomarkers of response to immunotherapy and describe how tumour and immune cells co-evolve and influence each other's evolutionary trajectories. By examining these interactions, we aim to uncover new biological targets associated with immunotherapy resistance. If validated through functional studies (in collaboration with Melcher and Arwert), these targets may pave the way for the development of innovative immunotherapeutic strategies.
Note: the ICR’s standard minimum entry requirement is a relevant undergraduate Honours degree (First or 2:1).
Pre-requisite qualifications of applicants:
- Candidates must have a First or 2:1 Honours degree or a Masters degree in computational biology, quantitative biology, computer science, statistics, or a related discipline.
- Demonstrable previous experience in data analysis using R and/or Python is essential.
- Previous experience with cancer/biological datasets desirable but not essential.
Intended learning outcomes:
- Deep knowledge of breast cancer genomics
- Knowledge of next-generation sequencing technologies, molecular pathology and molecular diagnostics.
- Skills in the quantitative analysis of bulk and single cell sequencing data
- Skills in the application of machine learning to biomedical data
- Scientific writing and presentation skills
[1] Barry, P., Vatsiou, A., Spiteri, I., Nichol, D., Cresswell, G.D., Acar, A., Trahearn, N., Hrebien, S., GarciaMurillas, I., Chkhaidze, K., Ermini, L., Huntingford, I.S., Cottom, H., Zabaglo, L., Koelble, K., Khalique, S., Rusby, J.E., Muscara, F., Dowsett, M., Maley, C.C., Natrajan, R., Yuan, Y., Schiavon, G., Turner, N., Sottoriva, A., 2018. The Spatiotemporal Evolution of Lymph Node Spread in Early Breast Cancer. Clin. Cancer Res. 24, 4763–4770. https://doi.org/10.1158/1078-0432.CCR-17-3374
[2] De Mattos-Arruda, L., Sammut, S.-J., Ross, E.M., Bashford-Rogers, R., Greenstein, E., Markus, H., Morganella, S., Teng, Y., Maruvka, Y., Pereira, B., Rueda, O.M., Chin, S.-F., Contente-Cuomo, T., Mayor, R., Arias, A., Ali, H.R., Cope, W., Tiezzi, D., Dariush, A., Dias Amarante, T., Reshef, D., Ciriaco, N., Martinez-Saez, E., Peg, V., Ramon Y Cajal, S., Cortes, J., Vassiliou, G., Getz, G., Nik -Zainal, S., Murtaza, M., Friedman, N., Markowetz, F., Seoane, J., Caldas, C., 2019. The Genomic and Immune Landscapes of Lethal Metastatic Breast Cancer. Cell Rep. 27, 2690-2708.e10. https://doi.org/10.1016/j.celrep.2019.04.098
[3] Liu, T., Liu, C., Yan, M., Zhang, L., Zhang, J., Xiao, M., Li, Z., Wei, X., Zhang, H., 2022. Single cell profiling of primary and paired metastatic lymph node tumors in breast cancer patients. Nat. Commun. 13, 6823. https://doi.org/10.1038/s41467-022-34581-2
[4] Reticker-Flynn, N.E., Zhang, W., Belk, J.A., Basto, P.A., Escalante, N.K., Pilarowski, G.O.W., Bejnood, A., Martins, M.M., Kenkel, J.A., Linde, I.L., Bagchi, S., Yuan, R., Chang, S., Spitzer, M.H., Carmi, Y., Cheng, J., Tolentino, L.L., Choi, O., Wu, N., Kong, C.S., Gentles, A.J., Sunwoo, J.B., Satpathy, A.T., Plevritis, S.K., Engleman, E.G., 2022. Lymph node colonization induces tumor-immune tolerance to promote distant metastasis. Cell 185, 1924-1942.e23. https://doi.org/10.1016/j.cell.2022.04.019
[5] Rueda, O.M., Sammut, S.-J., Seoane, J.A., Chin, S.-F., Caswell-Jin, J.L., Callari, M., Batra, R., Pereira, B., Bruna, A., Ali, H.R., Provenzano, E., Liu, B., Parisien, M., Gillett, C., McKinney, S., Green, A.R., Murphy, L., Purushotham, A., Ellis, I.O., Pharoah, P.D., Rueda, C., Aparicio, S., Caldas, C., Curtis, C., 2019. Dynamics of breast-cancer relapse reveal late-recurring ER-positive genomic subgroups. Nature 567, 399–404. https://doi.org/10.1038/s41586-019-1007-8
[6] Sammut, S.-J., Crispin-Ortuzar, M., Chin, S.-F., Provenzano, E., Bardwell, H.A., Ma, W., Cope, W., Dariush, A., Dawson, S.-J., Abraham, J.E., Dunn, J., Hiller, L., Thomas, J., Cameron, D.A., Bartlett, J.M.S., Hayward, L., Pharoah, P.D., Markowetz, F., Rueda, O.M., Earl, H.M., Caldas, C., 2022. Multi-omic machine learning predictor of breast cancer therapy response. Nature 601, 623–629. https://doi.org/10.1038/s41586-021- 04278-5
[7] Sammut, S.-J., Galson, J.D., Minter, R., Sun, B., Chin, S.-F., De Mattos-Arruda, L., Finch, D.K., Schätzle, S., Dias, J., Rueda, O.M., Seoane, J., Osbourn, J., Caldas, C., Bashford -Rogers, R.J.M., 2024. Predictability of B cell clonal persistence and immunosurveillance in breast cancer. Nat. Immunol. 25, 916– 924. https://doi.org/10.1038/s41590-024-01821-0
[8] Schmid, P., Cortes, J., Pusztai, L., McArthur, H., Kümmel, S., Bergh, J., Denkert, C., Park, Y.H., Hui, R., Harbeck, N., Takahashi, M., Foukakis, T., Fasching, P.A., Cardoso, F., Untch, M., Jia, L., Karantza, V., Zhao, J., Aktan, G., Dent, R., O’Shaughnessy, J., 2020. Pembrolizumab for Early Triple-Negative Breast Cancer. N. Engl. J. Med. 382, 810–821. https://doi.org/10.1056/NEJMoa1910549