Science Writing Prize 2024 - Back to the future: How new approaches could revolutionise old cancer treatments
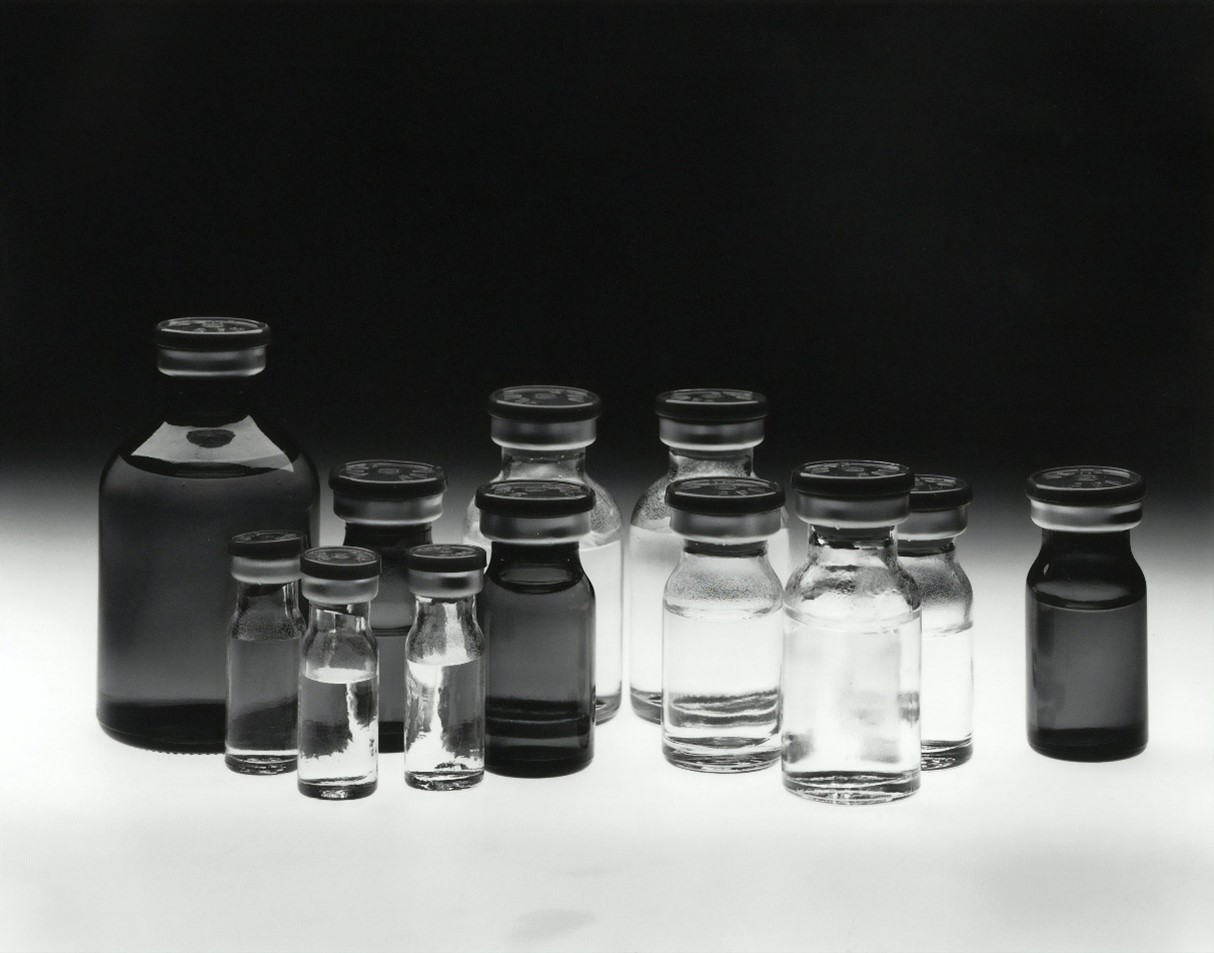
Chemotherapy might be old news, but the right approaches could dramatically improve their clinical usefulness. Credit: National Cancer Institute (via Unsplash)
Around the time the Human Genome Project was completed, there was considerable optimism for the future of healthcare. Grand claims were made. Time magazine declared in July 2000 that we would have “precise genetic definitions that make diagnosis sure and treatment swift”. Twenty years on, we are still waiting, although the collective understanding of the biological complexity of cancers has improved. (Mostly, we understand that cancers are far more complex than originally thought.)
Nowadays, these “genetic definitions” might be called biomarkers, broadly defined as “any measurable indicator of a biological process or condition”. Biomarkers come in many forms. Diagnostic biomarkers confirm the presence of disease, predictive biomarkers assess how effective a treatment might be, and prognostic biomarkers look at how long a patient might live. Biomarker research has exploded in recent years, but few ever become available as clinical tests. (Success rates may be as low as 1%). This phenomenon is so well-known that it even has a name: the “first translational gap”.
Bridging the gap
The first translational gap exists for many reasons. Tests to detect biomarkers need to be compatible with established hospital techniques. This is challenging because samples tend to be of lower quality and in lower quantities than research specimens (often due to the chemicals used to preserve tumour samples for long periods). To be useful, they also need to provide results in a timely manner – often within days or hours – and in an easily interpretable format. This is a tall order.
Revisiting old treatments
Biomarker research is undoubtedly progressing. Meanwhile, though, new cancer diagnoses in the UK have been rising year on year since the 1990s. This trend is forecast to continue into the 2030s and beyond. Patients diagnosed today do not have the luxury of waiting until the scientific community discovers biomarkers of the science-fiction calibre that Time magazine imagined. What should we do for them?
The solution might lie in an unlikely place. Cancer treatment has historically involved so-called “cut, burn, poison” methods: surgery to “cut” out the tumour, chemotherapy to “poison” with chemicals and radiotherapy to “burn” with radiation. Each has the potential to cure a patient’s cancer and extend their life, but each comes with potentially life-threatening side-effects. Decision-making in clinical practice relies on balancing risk and benefit, which is challenging when neither is quantified. If it were possible to identify which patients would benefit, oncologists could target treatments to these individuals whilst sparing others from unnecessary side-effects. In short, it would supercharge clinical pipelines.
A model approach
In principle, a patient’s likelihood of survival is affected by many things, including patient demographics (their age and sex, for example) and disease characteristics (such as the location and size of their tumour). Clinical factors like these could be used to build statistical models which identify patients with more aggressive disease.
Disease severity is only half the story, though. Identifying an aggressive cancer is useful, but it does not necessarily relate to whether it will respond to treatment. An oncologist needs to know the additional benefit of one treatment over another. There are many possible combinations of traditional treatments: surgery alone, surgery-plus-chemotherapy, and so on. Quantifying additional benefits would allow pinpointing of the most effective treatments. It would facilitate doctor-patient discussions around treatment approaches and help informed decision-making.
Fact or fiction?
The main difference between statistical models and biomarker tests lies in how easy they are to roll out to hospitals. Whilst biomarker tests require additional time and money, clinical factors are available as part of routine cancer diagnosis, and with no additional investment. Without the need for infrastructure investment, implementing statistical tools is far easier. And after all, tools are only beneficial if they are actually used.
This might sound like just another brand of science-fiction. But these models already exist. Some are in widespread use. Take PREDICT (https://breast.v3.predict.cam/), for example. It uses clinical factors to calculate the chance of death from breast cancer. Crucially, it also quantifies the additional benefit of giving various treatment combinations and presents the results in an easy-to-digest format ideal for facilitating discussions between doctors and patients. PREDICT has been evaluated by scientists all over the world and is endorsed by expert bodies such as the American Joint Committee on Cancer. It is freely available and receives around 30,000 online visits monthly from across the globe.
The future is now
PREDICT is nothing short of a huge success. To be able to quantify the benefit of treatment and target it to patients who will benefit most: that would have seemed like fantasy in the 1990s. The best part is that PREDICT’s approach is generalisable. It is possible to apply this to rare cancers, whose biology tends to be poorly understood. For example, sarcomas, a rare group of cancers which affect connective tissues like muscle and fat, are notoriously resistant to chemotherapy. Only 30% of patients gain any benefit; but knowing who these individuals are – using similar statistical models – would be revolutionary.
Models like PREDICT also provide a benchmark for comparison. How else would we know if a new biomarker is any good? By implementing similar models for every cancer, a standard would be set. Each challenger could be weighed against this gold standard to determine whether it is worth taking on. (It doesn’t need to be one-or-the-other, either: it is possible to incorporate effective biomarkers into these models to improve predictions further.)
It is tempting, when thinking about “treating cancers more precisely”, to imagine one-of-a-kind tailored drugs or “precise genetic definitions” like those from the turn of the millennium. Personalised medicine is undoubtedly coming; but that should not blind us to the opportunities that already exist. It is natural to be excited by the promises of tomorrow, but we must remember to do what we can today. Patients will thank us for it.
This piece was highly commended in the 2024 Mel Greaves Science Writing Prize.
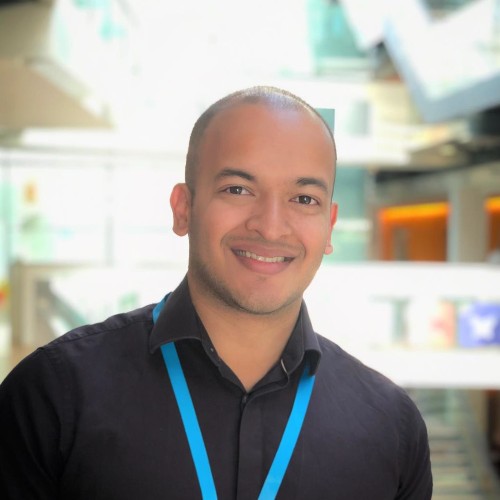
Dr Avirup Chowdhury is a public health doctor and ICR-Imperial Convergence Science clinical fellow. He joined the ICR in 2022 as part of Professor Paul Huang’s Molecular & Systems Oncology team, where his PhD focuses on investigating treatment response in soft tissue sarcoma.
Prior to joining the ICR, he studied medicine at the University of Glasgow and completed foundation training in Scotland. He then studied an MSc in Human Molecular Genetics at Imperial College London, before joining public health specialty training in the East of England and completing an MPhil in Public Health at the University of Cambridge.
His research interests include generating biological insights through deep molecular profiling, and translating these insights into statistical models which integrate molecular and clinical data to improve predictions in cancer.