Cancer Dynamics Group
Dr Stephen John Sammut’s group is developing computational and experimental frameworks that model changes in tumour ecosystem biology during treatment to inform optimal therapy choice in the next generation of personalised precision cancer medicine trials.
Research, projects and publications in this group
We are on a mission to unravel the complex biological mechanisms driving response and resistance to cancer treatments. Our group is deeply integrated with the Breast Unit at the Royal Marsden Hospital, one of the world's leading centres for cancer research and treatment.
Dr Stephen-John Sammut
Group Leader:
Cancer Dynamics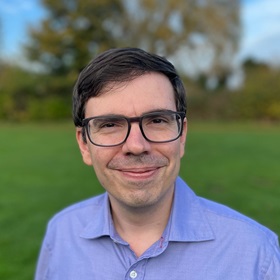
Dr Stephen John Sammut is a Clinician Scientist at the ICR and an Honorary Consultant in Medical Oncology at the Royal Marsden. He leads the Cancer Dynamics Group which uses high dimensional multiplatform tumour profiling to understand the biology underlying response to anticancer treatments.
Researchers in this group
Dr Stephen-John Sammut's group have written 37 publications
Most recent new publication 2/2025
See all their publicationsOur mission
At our laboratory, we are on a mission to unravel the complex biological mechanisms driving response and resistance to cancer treatments. By using machine learning to integrate data from multiple high-resolution cancer ecosystem profiling techniques, we aim to gain a deep understanding of how tumours and their surrounding cells behave and change when exposed to anticancer therapies. We are particularly interested in how these evolutionary trajectories are linked to treatment response and patient survival. With this knowledge, we aim to design the next generation of personalised precision medicine trials for breast cancer patients, ultimately improving outcomes for those affected by this disease.
Our translational multidisciplinary laboratory is deeply integrated with the Breast Unit at the Royal Marsden Hospital, one of the world's leading centres for cancer research and treatment. This prestigious association allows us to work with some of the top experts in the field of breast cancer research, giving us access to cutting-edge treatments, technologies, and expertise.
The inability to accurately predict response to treatment is a major unmet need
We are interested in optimising therapies for breast cancer that are given before surgery (known as neoadjuvant therapies). These types of therapies are routinely used in patients with higher risk early and locally advanced breast cancer and have several potential benefits. For example, they can shrink the size of the tumour, making it easier to remove surgically. They also reduce the likelihood of the cancer spreading to other parts of the body. Clinical trials have also shown that the degree of response to neoadjuvant therapy is strongly associated with survival.
Approximately 30% of patients treated with neoadjuvant therapies are found to have no residual cancer at the time of surgery, indicating that the treatment was successful. Most of these patients are cured. However, in 55% of cases, extensive cancer remains after treatment, indicating that the therapy was not as effective. These patients are at a higher risk of poorer outcomes and worse survival (Figure 1).
This high therapy failure rate is due in part to the fact that anticancer therapies are selected using empirical risk stratification models that predominantly consider clinical features and do not account for the molecular complexity of the tumour ecosystem (Figure 1). We are currently unable to provide personalised predictions of response to therapy to patients with breast cancer in the clinic and consequently continue to adopt ‘one size fits many’ approaches. This is a major unmet need: therapies should be delivered towards a predicted response, and patients with tumours predicted to be resistant to standard therapies should be recruited to clinical trials in which drugs that exploit tumour-specific vulnerabilities are explored.
Figure 1: The implementation of personalised precision breast cancer medicine in the clinic has been slow. As a result, breast cancer therapy selection is not optimised to the molecular profile of the tumour ecosystem and consequently many receive ineffective therapies.
Our laboratory has extensive expertise in the molecular profiling of early and metastatic breast cancer and we have contributed to the molecular reclassification of breast cancer, the deep characterisation of its mutational and methylation landscapes and the development of dynamic models that predict relapse and survival.
1. Investigating the biological mechanisms underlying treatment response and predicting how patients will respond to therapy
We have characterised the biological processes associated with response to chemo- and targeted therapies in early breast cancer and developed the first machine learning framework that combines genomic, transcriptomic and digital pathology data from diagnostic cancer biopsies to predict response to therapy (Figure 2). Our research has shown that the amount of residual cancer remaining after neoadjuvant therapy is closely related to certain properties of the tumour ecosystem before treatment, such as its mutational and copy number profiles, levels of proliferation and immune infiltration, and the extent of T cell dysfunction and exclusion. The effectiveness of therapy depends on the overall characteristics of the tumour ecosystem, which can be predicted using data integration and machine learning. This major advance in personalised precision cancer medicine has now provided us with the technology to forecast response to therapy in patients before commencing treatment.
We are now using advanced techniques such as single-cell profiling, spatial phenotyping, immune repertoire profiling and imaging analysis to study the biology of response to neoadjuvant therapies at a much higher resolution than ever before. By examining the tumour ecosystem using multiple technologies and integrating this data using machine learning, we aim to gain a deeper understanding of complex tumour biology and improve our ability to predict how patients with breast cancer will respond to treatment. This will eventually allow us to ensure that the right drug is administered to the right person.
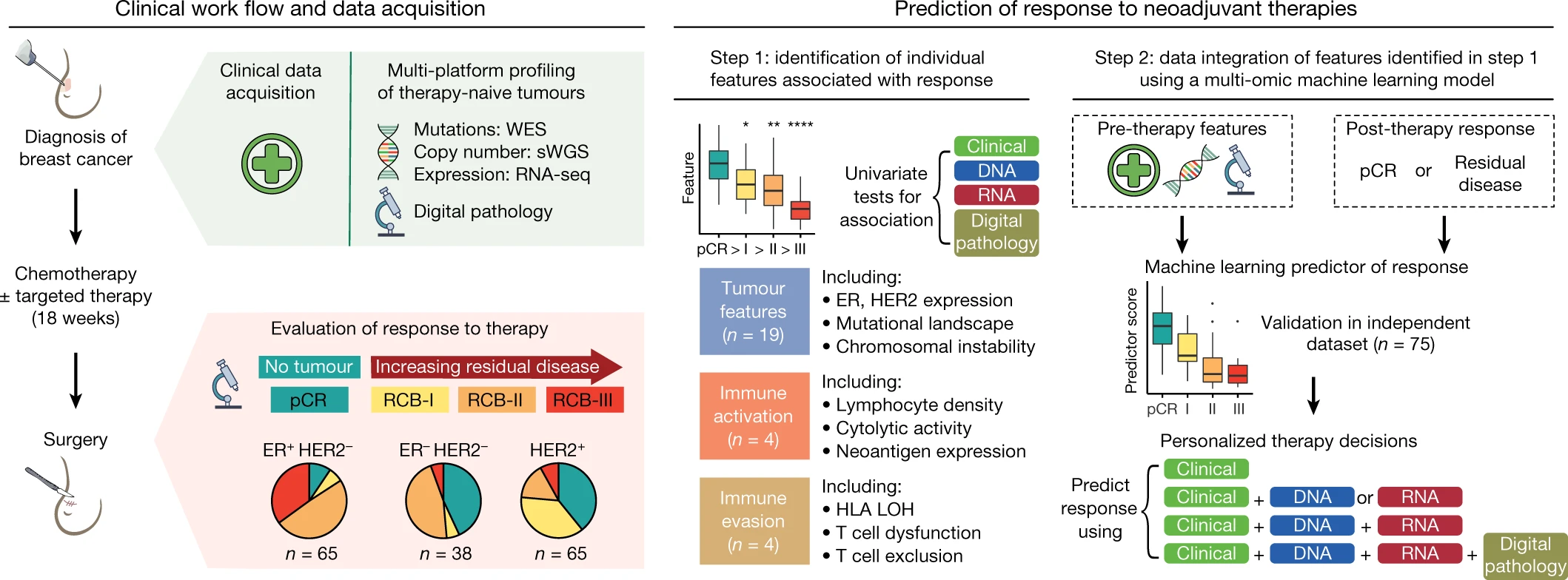
Figure 2: Predicting response to therapy using multi-platform profiling of therapy-naïve tumours and machine learning. Figure from Sammut, SJ. et al. Multi-omic machine learning predictor of breast cancer therapy response. Nature 601, 623–629 (2022).
2. Developing personalised dynamic biomarkers of response to therapy
We are now building on our published work and developing dynamic biomarker technologies that can track changes in tumour and microenvironment biology over time during neoadjuvant therapy. By profiling tumours in vivo at multiple time points during therapy using high-resolution molecular (such as single-cell sequencing, spatial phenotyping) and imaging (such as magnetic resonance imaging and digital pathology) technologies, we are studying how tumours and their ecosystems evolve and adapt during treatment and identifying how these evolutionary trajectories are related to the effectiveness of the therapy. Importantly, this will allow us to understand better the biology of chemoresistant disease and allow us to identify vulnerabilities that can be targeted.
We aim to use this knowledge to develop a clinically implementable predictor of response that integrates data from the pre and on-therapy tumour ecosystem to identify therapy failure early and accurately. This predictor will then be integrated into the next generation of personalised precision cancer medicine trials to inform early treatment switch if therapy failure is detected.