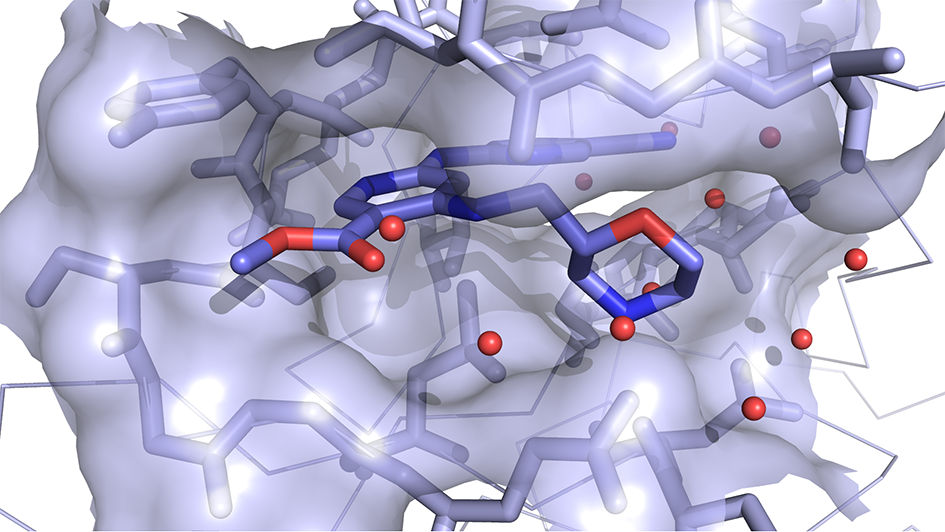
Designing new and better drugs in the fight against cancer is becoming increasingly challenging as we discover more about the disease itself and how it evolves over time in the body. We need to box clever in designing new drugs that do not solicit resistance and are engaged in an arms race between resilient cancer clones and the new therapies.
Most small molecule drugs are a collection of twenty or thirty atoms, typically carbon, nitrogen, oxygen, sulphur with a smattering of halogens like chlorine and fluorine, connected in a way that imparts biological functionality.
However, while this definition appears relatively simple and suggests that the number of possible drug-like molecules would be rather small, when we do the maths we end up with a vast number of possible molecules due to a phenomenon known as combinatorial explosion.
A simple analogy of drug design is building models with Lego bricks. Given only a few Lego bricks it is possible to make a seemingly endless number of different models.
Indeed, the foundations of this exploration of chemistry space goes back over a century to Arthur Cayley who used mathematics to enumerate possible chemical structures, what he called kenograms, from a given number of atoms.
With each additional atom in a molecule, the possible ‘space’ of potential drugs increases roughly ten-fold. This means that the space of all possible drug-like molecules reaches one million billion billion billion, or 1,000,000,000,000,000,000,000,000,000,000,000.
Clearly, even using computational methods, we need efficient ways of exploring and exploiting this vast potential drug space!
Prioritising the most promising potential drugs
We have to select more efficiently from the vast array of potential drugs that can be synthesised with a limit on the number that we can physically make and test. This is where computers can play a significant role in assisting drug designers and chemists to prioritise the most promising potential drugs to make, using many different modelling techniques.
At the ICR, we have developed algorithms to sample the possible chemistry space using analogues of evolutionary theory by selecting the best molecular structures entirely in the computer using artificial intelligence to predict the desirable properties.
Drug design is inherently a process that requires optimisation of multiple different properties. We often generate predictive models by using artificial intelligence to identify what drives the different properties according to the structures of the molecules that have already been made and tested.
These models can then be applied to new potential drugs to evaluate their suitability entirely within the computer, an approach called in silico optimisation. We also apply structural biology data, such as the known 3D binding sites of proteins implicated in the disease we aim to treat.
We can then simulate how the potential drugs may interact with protein binding sites by using algorithms that ‘dock’ to understand how they may interact with the proteins and score them appropriately. Combining all these modelled properties, and more besides, we are able to reliably score the new potential drugs as part of the in silico optimisation.
Using artificial intelligence models
The algorithms we have developed initially generate a population that samples the relevant potential drug space and then use computational analogues of recombination and mutation as in genetic evolution.
The molecular structures in the population are chopped up into fragments and those fragments used to populate a second generation, according to the ‘fitness’ of the parents using the artificial intelligence models above.
Additionally, the structures are randomly mutated – by, for example, adding or deleting atoms, and changing the elements, from carbon to nitrogen. This results in a second generation of drugs ready for evaluation against the optimisation criteria.
This process is re-iterated and rapidly evolves to the most optimal potential drugs according to the models of interest, globally exploring the space and locally exploiting the most interesting regions of suitability.
The In Silico Medicinal Chemistry Team in the Cancer Research UK Cancer Therapeutics Unit supports the activities of more than 50 PhD medicinal chemists working towards novel cancer treatments.
Read more
Making computerised predictions
Using computational models of evolutionary theory to optimise potential drugs against artificial intelligence models, our algorithms have been applied to a number of drug design projects at the ICR.
Our computationally designed potential drugs have helped our drug design project teams make better decisions and answer more questions with fewer prototype molecules.
Many of these potential drugs have progressed to the clinic demonstrating the effectiveness of modern computational and artificial intelligence methods in helping to design the next generation of effective anti-cancer drugs.
As artificial intelligence and genetic optimisations gain increased application in drug discovery the optimisation of potential drugs will become much more effective. We will begin to see artificial intelligence augmenting and assisting human intelligence and powering more effective decision-making.
This does not necessarily imply that we will need to make and test fewer potential drugs to find the clinical candidate, but it will certainly mean we will be making and testing the best choices throughout the process that will help us learn more about the drug space under investigation.
The wider application of these methods will also highlight the importance of large datasets and better quality data from which we can make these computerised predictions.
comments powered by