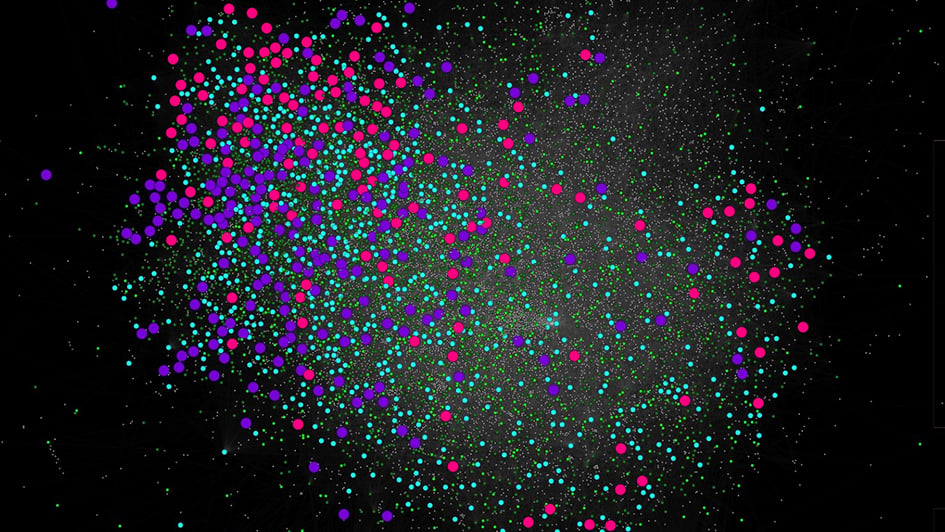
A Big Data cloud (image: Costas Mitsopoulos , Amanda C. Schierz , Paul Workman, Bissan Al-Lazikani)
Imagine if you could read all 3 million letters that make up the human genetic code. “Why would you want to?” you might ask.
Well, by finding the differences between the sequences of a cancer and a normal tissue sample, it will allow you to specifically identify the genetic changes that may have caused the tumour.
By sequencing a patient’s genome, this will also allow doctors to determine if a patient has a genetic change that can alter that person’s response to a particular treatment. For example, it could help assess how sensitive a person will be to the blood thinning drug, warfarin, allowing doctors to more accurately establish the best dose.
In 2003, sequencing a genome took 13 years and cost $2.7 billion. Since then, the price of sequencing has plummeted, particularly in the last five years, and it is now possible to perform this same process in a matter of days for a little over $1,000.
.png?sfvrsn=8d354269_0)
Costs of genome sequencing
With sequencing becoming so readily available, an emerging problem is how to store all the data. While the raw data from sequencing a human genome will fill up about four BluRay disks, the additional downstream analyses can take up to three times that. With all the human sequencing projects taking place, it has been estimated that by 2025, 2–40 exabytes (EB, 1 EB = 1 billion gigabytes) of storage capacity will be required. That’s more than even YouTube or Twitter will generate in the same time!
Data sizes of genome sequencing
OK, so we’re going to need lots of hard drives to store all this genetic data. However, this is only part of the puzzle. Doctors and researchers are becoming swamped with data from other sources, including patient information, lab test results, medical histories and imaging scans, such as CT or MRI scans. With the increasing popularity of wearable fitness trackers, such as the Apple Watch or Fitbit, the generation of medical data will become easier than ever. This is a vast resource to mine for improving the treatment of cancer.
Discoveries in science are driven by observing patterns in data – Darwin noticing the differences between finches in the Galapagos Islands contributed to his idea of natural selection; Mendel’s observations of inheritance patterns in peas led to modern genetics.
With the current enormous datasets, it’s increasingly difficult to find the patterns. Having worked in this field for several years, I’ve seen first hand the many changes and challenges that have appeared in just the last few years – from the immense growth in data, to the challenges in storing and accessing this data.
These vast volumes of data require new approaches to data analysis. Already, this problem has attracted the attention of a number of companies. The Intel Collaborative Cancer Cloud is attempting to bring together data from multiple hospitals and research centres so that data on how other patients with similar genetic profiles responded can be leveraged to personalise treatment.
Other examples include IBM using their computing system, Watson, to recommend a drug that it thinks is likely to treat a patient, or Google’s DeepMind, which in addition to its famous AlphaGo program, is using data from the NHS to improve patient outcomes.
But wait, there’s more! In addition to assisting in the treatment of patients, these large integrated datasets will also aid in the development of new drug targets.
With the increase in cost in the development of new drugs, any means which would mean faster development is essential. By investigating data from genetic studies, drug targets, scientific papers, and clinical trials, it is possible to identify new targets that can be used to treat cancer. It is also to apply a known drug to a different disease (‘repurpose' a drug). For example, there is some evidence that aspirin, a common pain medication, could be protective from colorectal cancer.
Costs of drug development
While this is all very exciting, I should also urge caution. These big data analyses are not a panacea to solving disease. More data also means more noise, so we need new questions and new ways of thinking about the data to utilise these datasets to their fullest extent. There are many challenges in integrating diverse datasets, and there is certainly much to be gained from using these datasets. The main problem is not to get overconfident.
Harold Varmus, former director of both the US National Institute of Health and the National Cancer Institute, won the Nobel Prize in Physiology or Medicine in 1989 for discovering proto-oncogenes – genes that can result in cancer if they become mutated. In his acceptance speech he said:
“We recognise that, unlike Beowulf at the hall of Hrothgar, we have not slain our enemy, the cancer cell, or figuratively torn the limbs from his body. In our adventures, we have only seen our monster more clearly and described his scales and fangs in new ways – ways that reveal a cancer cell to be, like Grendel, a distorted version of our normal selves. May this new vision… inspire our band of biological warriors to inflict much greater wounds tomorrow.”
We are in an exciting but challenging time in the analysis of biological data. I am certain that these data-driven analyses will provide the means to reveal further weaknesses in cancer and provide improved methods for treating it.
This article was shortlisted for the Professor Mel Greaves Science Writers of the Year 2016 prize, originally presented at the ICR annual conference in June 2016.
Dr Philip Law is a statistician/bioinformatician in the Genetics and Epidemiology division at the ICR.
comments powered by